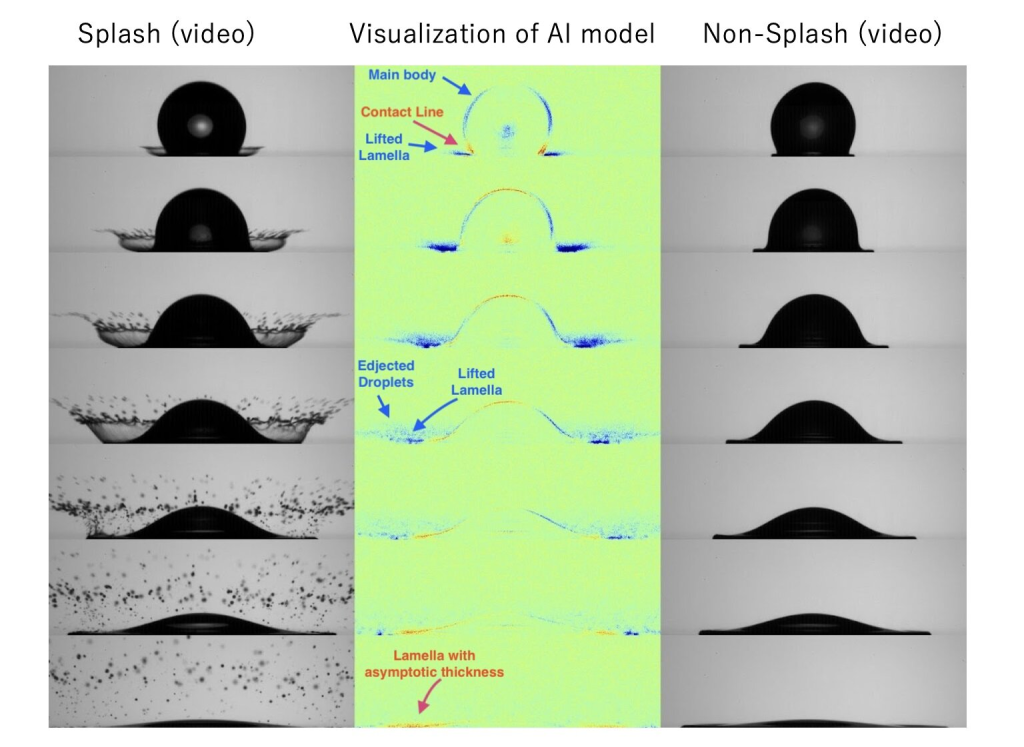
The impact of a drop on a solid surface is an important phenomenon that has various applications. Especially when the drop splashes, it can cause deterioration of printing and paint qualities, erosion, and propagation of airborne virus, among others. Therefore, it is important to observe and understand the characteristics of the splashing drops of different liquids.
However, the multiphase nature of the phenomenon causes difficulties for observation when it is performed merely using the naked eye. Although the recent development of artificial intelligence (AI) has shown promise in tackling this problem, AI models usually function as a black box, where the underlying decision-making process is unknown.
At the Tokyo University of Agriculture and Technology, a research team from the Department of Mechanical Systems Engineering has developed an explainable AI to observe and understand the splashing drops of different liquids from an AI perspective.
The research team led by Prof. Yoshiyuki Tagawa and Prof. Akinori Yamanaka, which includes Jingzu Yee (former assistant professor), Pradipto (former assistant professor), Shunsuke Kumagai (1st-year master’s student), and Daichi Igarashi (former master’s student), published their findings in Flow on December 20, 2024.
The research team adopted the architecture of a feedforward neural network to develop an AI model to classify videos of splashing and non-splashing drops that were recorded using a high-speed camera.
After training, the AI model has successfully classified videos of splashing and non-splashing drops with a success rate of 92% for low-viscosity liquid and 100% for high-viscosity liquid. Then, the researchers implemented their proposed method of visualizing the AI to analyze and interpret the classification process.
Their results show that the AI classifies splashing and non-splashing drops from the contour of the drop’s main body, the ejected droplets, and the thin sheet ejected from the side of the drop called lamella. Moreover, the proposed visualization method successfully determined which frame of the video has the most influence on the classification of the AI.
The results show that the differences between splashing and non-splashing drops of low-viscosity liquids are more obvious during the earlier stage of the impact, while it is more obvious during the later stage of the impact for high-viscosity liquids.
“Our newly proposed explainable AI method provides an alternative to the conventional investigation methods for drop impact research,” said Jingzu Yee, a former assistant professor at the Tokyo University of Agriculture and Technology.
“Our method reveals the fundamental aspects of drop impact, which can be leveraged to enable various devices and systems that will benefit humankind.”
More information: Jingzu Yee et al, Morphological evolution of splashing drop revealed by interpretation of explainable artificial intelligence, Flow (2024). DOI: 10.1017/flo.2024.28
Provided by Tokyo University of Agriculture and Technology