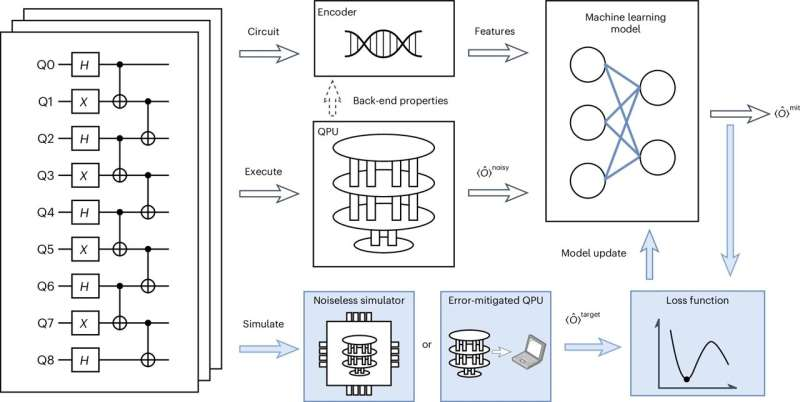
Quantum computers have the potential of outperforming classical computers in some optimization and data processing tasks. However, quantum systems are also more sensitive to noise and thus prone to errors, due to the known physical challenges associated with reliably manipulating qubits, their underlying units of information.
Engineers recently devised various methods to reduce the impact of these errors, which are known as quantum error mitigation (QEM) techniques. While some of these techniques achieved promising results, executing them on real quantum computers is often too expensive or unfeasible.
Researchers at IBM Quantum recently showed that simple and more accessible machine learning (ML) techniques could be used for QEM. Their paper, published in Nature Machine Intelligence, demonstrates that these techniques could achieve accuracies comparable to those of other QEM techniques, at a far lower cost.
“We started to think about how to depart from conventional physics-based QEM methods and whether ML techniques can help to reduce the cost of QEM so that more complex and larger-scale experiments could come within reach,” Haoran Liao, co-first author of the paper, told Phys.org.
“However, there seemed to be a fundamental paradox: How can classical ML learn what noise is doing in a quantum calculation running on a quantum computer that is doing something beyond classical computers? After all, quantum computers are of interest for their ability to run problems beyond the power of classical computers.”
As part of their study, the researchers carried out a series of tests, where they used state-of-the-art quantum computers and up to 100 qubits to complete different tasks. They focused on tasks that are impossible to complete via brute-force calculations performed on classical computers, but that could be tackled using more sophisticated computational methods running on powerful classical computers.
“This interesting ‘paradox’ is another motivation for us to think about whether careful designs can tailor ML models to find the complicated relationships between noisy and ideal outputs of a quantum computer to help quantum computations,” said Liao.
The primary objective of the recent study by Liao and his colleagues was to accelerate QEM using widely used ML techniques, demonstrating the potential of these techniques in real-world experiments. The team first started experimenting with a complex graph neural network (GNN), which they used to encode the entire structure of a quantum circuit and its properties, yet they found that this model performed not very well.
“It surprised us to find that a simpler model, random forest, worked very well across different types of circuits and noise models,” explained Liao.
“In our exploration of the ML techniques for QEM, we tried to shape and vary the noise to see how different techniques ML or conventional, perform in different scenarios, so we can better understand the capability of ML models in ‘learning’ the noise–we are not passive bystanders, but active agents.
“We not only tried to benchmark but also demystify the ‘black box’ nature of the ML models in the context of learning relationships between noisy and ideal outputs of a more powerful quantum computer. “

The experiments carried out by Liao and his colleagues demonstrate that ML could help to accelerate physics-based QEM. Remarkably, the model that they found to be most promising, known as random forest, is also fairly simple and could thus be easy to implement on a large scale.
“We assessed how much data is needed to train the ML models well to mitigate errors on a much larger set of testing data and clearly demonstrated a substantially lower overall overhead of the ML techniques for QEM without sacrificing accuracy,” said Liao.
The findings gathered by this team of researchers could have both theoretical and practical implications. In the future, they could help to enrich the present understanding of quantum errors, while also potentially improving the accessibility of QEM methods.
“Is the relationship between noisy and ideal outputs of a quantum computer fundamentally unlearnable? We didn’t know the answer,” said Liao.
“There are a lot of reasons why this would be impossible to determine. However, we showed this key relationship is in fact learnable by ML models in practice. We also showed that we are not passive bystanders, but we can shape the noise in the computation to improve the learnability further.”
Liao and his colleagues also successfully demonstrated that ML techniques for QEM are less costly, yet they can achieve accuracies comparable to those of alternative physics-based QEM techniques. Their experiments were the first to demonstrate the potential of machine learning algorithms for QEM at a utility scale.
“Even in the most conservative setting, ML-QEM demonstrates more than a 2-fold reduction in runtime overhead, addressing the primary bottleneck of error mitigation and a fundamentally challenging problem with a substantial leap in efficiency,” said Liao.
“Most conservatively, this translates into at least halving experiment durations—e.g., cutting an 80-hour experiment to just 40 hours—drastically reducing operational costs and doubling the regime of accessible experiments.”
After gathering these promising results, Liao and his colleagues plan to continue exploring the potential of AI algorithms for simplifying and upscaling QEM. Their work could inspire other research groups to conduct similar studies, potentially contributing to the advancement and future deployment of quantum computers.
“This is just the beginning, and we’re excited about the field of AI for quantum,” added Liao. “We would like to emphasize that at least in the context of QEM, ML is not to replace, but to facilitate physics-based methods, that they can build off each other.
“This opens the door for what’s possible with ML in quantum and is an invitation to the community that ML can perhaps accelerate and improve many other aspects of quantum computations.”
More information: Haoran Liao et al, Machine learning for practical quantum error mitigation, Nature Machine Intelligence (2024). DOI: 10.1038/s42256-024-00927-2.
Journal information: Nature Machine Intelligence
© 2024 Science X Network