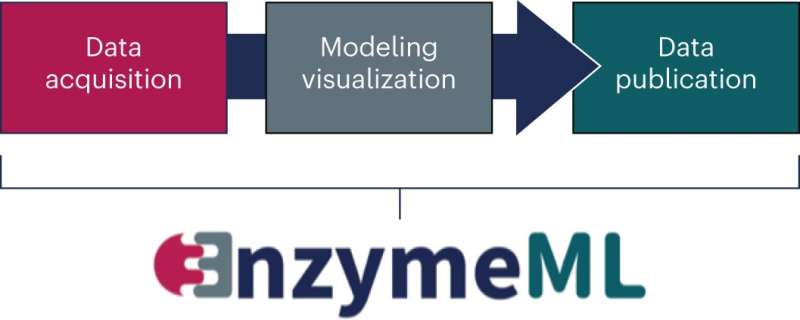
In catalytic sciences, as in all scientific fields, we face a rapidly increasing volume and complexity of research data, which is a challenge for analysis and reuse. A team led by Prof. Jürgen Pleiss from the Institute of Biochemistry and Technical Biochemistry at the University of Stuttgart has introduced EnzymeML as a data exchange format in a recent journal article published in Nature Methods. EnzymeML serves as a format to comprehensively report the results of an enzymatic experiment and stores the data in a structured way to make it traceable and reusable.
While more and more data is generated by an increasing number of researchers and research expenditures increase worldwide, this data is hardly manageable by the standard scholarly practice of communicating scientific results. Even managing your own data manually is time-consuming and error-prone, but accessing and re-analyzing data from other research groups is almost impossible. The lack of standards, incomplete metadata, and missing original data make it nearly impossible to reproduce published results. More and more researchers feel like they are drowning in a tsunami of data.
This also applies to studies on the catalytic activity, selectivity and stability of enzymes and enzymatic networks, a field of research that is equally important for industrial biotechnology and biomedicine. What also complicates matters in this area is the fact that data describing enzymatic experiments is particularly complex, because an enzymatic reaction depends on many factors, such as the protein sequence of the enzyme, the recombinant host organism, the reaction conditions, and non-enzymatic secondary reactions. Furthermore, other effects such as inactivation or inhibition of the enzyme or evaporation of the medium affect the results.
The new, standardized data exchange format EnzymeML, presented by 23 authors from 14 different research institutions in the journal Nature Methods addresses this dilemma. EnzymeML can completely record the results of an enzymatic experiment, from the reaction conditions to the measured data, as well as the kinetic model used to analyze experimental data and the estimated kinetic parameters. The format thus provides a seamless communication channel between experimental platforms, electronic lab notebooks, enzyme kinetics modeling tools, publication platforms, and enzymatic reaction databases.
“We demonstrate the feasibility and usefulness of the EnzymeML toolbox using six scenarios where data and metadata from various enzymatic reactions is collected, analyzed, and uploaded to public databases for future use,” explains first author Simone Lauterbach.
EnzymeML documents are structured and standardized, therefore the experimental results encoded in an EnzymeML document are interoperable and reusable by other groups. Because an EnzymeML document is machine-readable, it can be used in an automated workflow to store, visualize, and analyze data, as well as reanalyze previously published data, with no restrictions of the size of each data set, or the number of experiments.
“The digitalization of biocatalysis increases the efficiency of data management, visualization and analysis,” says Prof. Jürgen Pleiss, corresponding author, and project coordinator. Furthermore, digitalization improves the reproducibility of experiments and data analyses, thus promoting trust in scientific results. “The EnzymeML toolbox makes best use of rapidly growing enzymatic data and is a useful tool that allows researchers to surf the research data wave.”
More information: Simone Lauterbach et al, EnzymeML: seamless data flow and modeling of enzymatic data, Nature Methods (2023). DOI: 10.1038/s41592-022-01763-1
Journal information: Nature Methods
Provided by University of Stuttgart