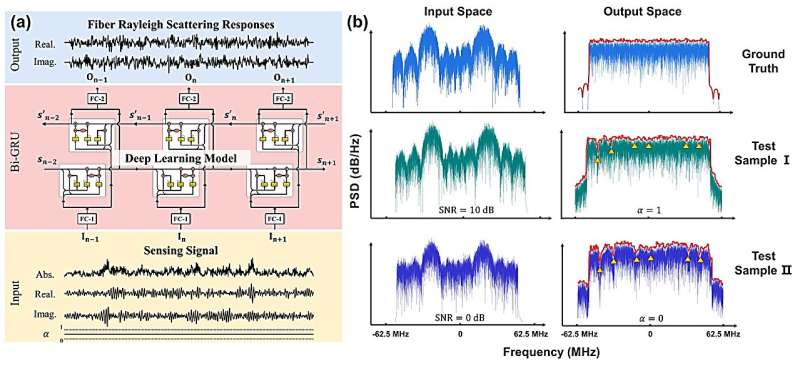
Scattering in media has unique inherent randomness. Fiber Rayleigh scattering is one of the typical scattering effects. The exploration and understanding of the characteristics of fiber Rayleigh scattering holds significant research value for optical fiber sensing, random fiber lasers, etc.
However, in practical noisy environments, accurately extracting the fiber Rayleigh scattering is a challenging issue. If deep learning models can express the general characteristics of fiber Rayleigh scattering and be trained by numerical simulation datasets only, it will have significant scientific and practical value.
The general characteristics of fiber Rayleigh scattering based on a deep learning model were successfully extracted for the first time by a research group from UESTC. Published in Science China Information Sciences, the research utilized a purely numerical simulation dataset to train a self-built deep learning model, to extract the general characteristics of fiber Rayleigh scattering.
The experimental verification was then carried out by a widely-used distributed acoustic sensing system known as the phase-sensitive optical time domain reflectometry (Φ-OTDR). The specific innovations and significance are as follows:
1. By training with a numerical simulation dataset, the deep learning model successfully captures the general characteristics of fiber Rayleigh scattering, and accurately recovers the broadband fiber Rayleigh scattering based on narrowband sensing signal in the experiment. This model has strong generalization ability, and will not overly rely on the data generated under specific experimental conditions.
Therefore, it is possible to use numerical simulation to construct large-scale and diverse training datasets for Φ-OTDR systems and train multi-functional artificial intelligence based on complex models.
2. With the fiber Rayleigh scattering obtained by the deep learning model, the optimal probe pulse can be estimated beforehand, and then the time-domain responses of the sensing signal can be optimized, achieving adaptive waveform modulation in the Φ-OTDR system. This opens up new ideas for other research directions based on Rayleigh scattering optical systems, such as random fiber laser, adaptive optics with wavefront correction, etc.
This work investigates the utilization of a deep learning model for extracting the characteristics of Rayleigh scattering in optical fibers. The discoveries provide a basis for developing a multi-functional artificial intelligence specifically designed for distributed optical fiber sensing and also offer valuable insights for other optical systems based on Rayleigh scattering.
More information: Yongxin Liang et al, Prediction of fiber Rayleigh scattering responses based on deep learning, Science China Information Sciences (2023). DOI: 10.1007/s11432-022-3734-0. www.sciengine.com/SCIS/doi/10. … 4b-bd8f-6fe346fa3db8
Provided by Science China Press