by Ingrid Fadelli , Phys.org
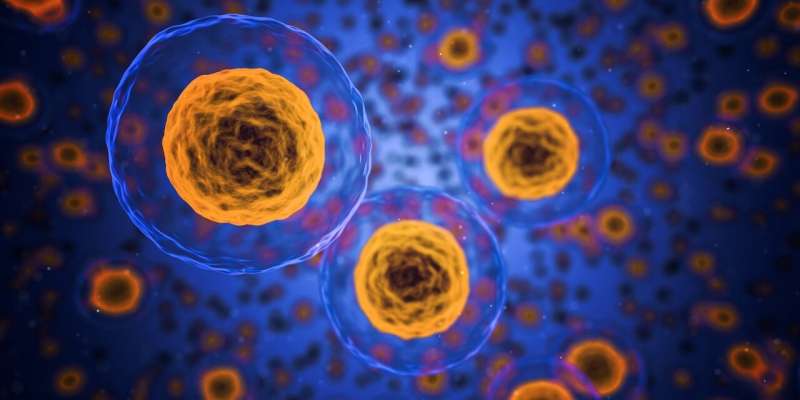
The behaviors, physiology and existence of living organisms is supported by countless biological processes, which entail the communication between cells and other molecular components. These molecular components are known to transmit information to each other in various ways, for instance via processes know as diffusion and electrical depolarization or by exchanging mechanical waves.
Researchers at Yale University recently carried out a study aimed at calculating the energetic cost of this transfer of information between cells and molecular components. Their paper, published in Physical Review Letters, introduces a new tool that could be used to study cellular networks and better understand their function.
“We have been thinking about this project for a while now in one form or another,” Benjamin B. Machta, one of the researchers who carried out the study, told Phys.org.
“I first discussed ideas that eventually morphed into this project with my Ph.D. advisor Jim Sethna about a decade ago, but for various reasons that work never quite took off. Sam and I started talking about this when thinking about how to understand the energy costs that biology needs to spend to compute—a theme in much of his Ph.D. work- and maybe more broadly to ensure its parts are coherent and controlled, and he figured out how to do these calculations.”
The recent work by Machta, and his colleague Samuel J. Bryant draws inspiration from earlier papers published in the late 90s, particularly efforts by Simon Laughlin and his collaborators. At the time, this research group had tried to experimentally determine how much energy neurons spend when sending information.
“Laughlin and colleagues found that this energy expenditure ranged between 104-107 KBT/bit depending on details, which is far higher than the ‘fundamental’ bound of ~ KBT/bit, sometimes called the the Landauer bound which must be paid to erase a bit of information,” Machta explained.
“In some ways we wanted to understand; was this an example of biology just being wasteful? Or maybe there were other costs that needed be paid; in particular, the Landauer limit makes no reference to geometry or physical details. Applying the Landauer bound is itself subtle, because it is only paid on erasing information it is possible to compute reversibly, never erase anything, and not pay ANY computing cost- but that is not the focus here.”
A further objective of the recent study by Machta and Bryant was to determine whether optimizing these energetic costs could shed light on the reasons why molecular systems communicate with each other using distinct physical mechanisms in different situations. For instance, while neurons typically communicate with each other via electrical signals, other types of tells can communicate via the diffusion of chemicals.
“We wanted to understand in what regime each of these (and others) would be best in terms of an energy cost per bit,” Machta said. “In all our calculations, we consider information that is sent through a physical channel, from a physical sender of information (like a ‘sending’ ion channel that opens and closes to send a signal) to a receiver (a voltage detector in the membrane which could also be an ion channel). The heart of the calculation is a textbook calculation for the information rate through a Gaussian channel, but with a few new twists.”
Firstly, in their estimations, Machta and his colleagues always consider a physical channel, in which currents of physical particles and electrical charges are carried according to a cell’s physics. Secondly, the team always assumed that a channel is corrupted by thermal noise in the cellular environment.
“We can calculate the spectrum of this noise with the ‘fluctuation dissipation theorem’ which relates the spectrum of thermal fluctuations to the near equilibrium response functions,” Machta explained.
A further unique feature of the team’s estimations is that they were performed using relatively simple models. This allowed them the researchers to always place conservative lower bounds on the energy required to power a channel and drive physical currents in a biological system.
“Because the signal must overcome thermal noise, we generally find costs with a geometric prefactor multiplying “KBT/bit,'” Machta said.
“This geometric factor can have the size of the sender and receiver; a large sender generally decreases costs per bit by allowing a dissipative current to be spread over a larger area. Moreover, a larger receiver allows more averaging over thermal fluctuations, so that a weaker overall signal can still carry the same information.”
“So, for example, for electrical signaling, we get a form for the cost per bit that scales like r2/σI σO kBT/bit, where r is the distance between sender and receiver and σI,σO are the size of the sender and receiver. Importantly, for ion channels which are a few nanometers across, but which send information over microns, this cost could easily be many orders of magnitude larger than kT/bit that simpler (or more fundamental) arguments suggest as a lower bound.”
Overall, the calculations performed by Machta and his colleagues confirm the high energetic cost associated with the transfer of information between cells. Ultimately, their estimations could be the beginning of an explanation for the high cost of information processing measured in experimental studies.
“Our explanation is less ‘fundamental’ than the Landauer bound, in that it depends on the geometry of neurons and ion channels, and other details,” Machta said. “However, if biology is subject to these details, then it may be that (for example) neurons are efficient and up against real information/energy limitations, and not merely inefficient. These calculations are certainly not enough to yet say that any particular system is efficient, but they do suggest that sending information through space can necessitate very large energy costs.”
In the future, this recent work by Machta and his colleagues could inform new interesting biological studies. In their paper, the researchers also introduced a ‘phase diagram,” representing situations in which the selective use of specific communication strategies (e.g., electrical signaling, chemical diffusion, etc.) is optimal.
This diagram could soon help to better understand the design principles of different cell signaling strategies. For instance, it could shed light on why neurons use chemical diffusion to communicate at synapses, but they use electrical signals when sending information over hundreds of microns from dendrites to the cell body; as well as why E. coli bacteria utilize diffusion to send information about their chemical environment.
“One thing we are working on now is trying to apply this framework towards understanding the energetics of a concrete signal transduction system,” Machta added.
“Our recent work just considered the abstract cost of sending information between two single components—in real systems there are typically information processing networks, and applying our bound requires understanding the flow of information in these networks. This goal also comes with new technical issues—applying our calculations to specific geometries (like a ‘spherical’ neuron or an axon which resembles a tube, each importantly different than the infinite plain we used here).”
More information: Samuel J. Bryant et al, Physical Constraints in Intracellular Signaling: The Cost of Sending a Bit, Physical Review Letters (2023). DOI: 10.1103/PhysRevLett.131.068401
Journal information: Physical Review Letters
© 2023 Science X Network